My initial thought was to begin this article with the traditional sob story that every last place, self-absorbed fantasy owner sings; “Everyone on my team got hurt, all my stars underperformed, and I played every team in my league on their best week.”
While all of this may be true for my 12 team head-to-head categories league team, I pretense this article with the statement above, not to gain sympathy, but to give context to the research that resulted in this article.
In this article I show what metrics have the highest correlation with the fantasy basketball player rater score and propose a linear regression that looks at an expected player rater score based off of the variables with the highest correlations.
All of the references to player rater scores in this article refer to the player rater scores that are produced by ESPN Fantasy Basketball for 12 team head to head category leagues that use these categories: points, rebounds, three pointers, field goal percentage, free throw percentage, blocks, and steals.
The population for this study looks at player rater scores from the 2015 season so far. So while the sample size is very small, it gives us a general look at what metrics, outside of the metrics that are categories themselves, correlate highly to player rater scores. I was also unable to find a centralized database that archived historical player rater data, or any value metric in general, for fantasy basketball. I also didn’t have the time to go through each season from the last decade, create z scores for each category at each position, and create the player rater scores myself. But if anyone knows where I can get my hands on this information, I would be happy to do a more conclusive study.
We also don’t want to look at player rater scores specifically; we want to look at player rater score per game. This allows for us to give credit to players like Russell Westbrook and Chris Bosh, who have been absent from play for an appreciable duration of the season, but have played exceptionally well while they’ve been on the court.
Bellow are the metrics that I looked at and their correlation to player rater score per game:
[table id=83 /]
The two numbers that immediately jump out are MP (minutes played) and TOV (turnovers); all of the other metrics ahead of these statistics can be discounted because they are numbers that directly contribute to one of the eight categories used in ESPN head to head category leagues.
The .672 r for minutes played and the .558 r for turnovers show a strong positive relationship with player rater score per game and are statistically significant numbers.
A week ago, before I ran a correlation on all of the metrics above, I just looked at minutes played and usage rate as stats that might correlate highly with player rater scores. To me these numbers made the most sense; minutes per game shows that you are able to get on the court enough to get the opportunity to produce, and usage rate shows that when you get the opportunity, your team incorporates you in what they do from a schematic sense.
It may be because of the small sample of player seasons that was used for this study, but it looks like minutes played and turn overs are better proxies for opportunity and incorporation than minutes per game and usage rate.
I’m still not sure why minutes played correlates more highly with player rater score per game than minutes played per game (I’d love to hear what any readers might think), but my theory is that a player of lesser skill can artificially inflate his minutes played per game by playing big minutes in blow outs or games where players ahead of him on the depth chart are hurt or in foul trouble.
Turnovers make more sense. Think about it this way. Players that get a lot of turnovers are just like guys that get caught cheating on their girlfriends multiple times and don’t get broken up with; there has to be some aspect of who they are that makes them redeeming enough to keep the girlfriend around for more; that or their girlfriend has daddy issues, low self esteem, and is in her mid thirties and is afraid that she won’t be able to find another man to start a family with—either way. In order to get a large volume of turnovers, you have to be good enough to leave you in the game after you’ve made a mistake and continue to take risks. Just like Karen let Hank Moody back into her life again and again, after mistake upon mistake, Karen, just like the team, may have thought it was worth the risk.
[youtube http://www.youtube.com/watch?v=7G3Vex09TKY?rel=0]
Below is a linear regression of expected player rater score per game, with player rater score per game as the dependent variable and minutes played and turnovers as the independent variables.
[table id=86 /]
xPR/GM produces an r of .714 with this formula: xPR/GM =- 0.2063 + 0.0003 * MP + 0.0016 * TOV.
While I was able to produce a linear regression with minutes played per game and usage rate that yielded a correlation of .687, it appears that minutes played and turnovers are much better proxies for our formula.
I’m not sure there is much to xPR/GM other than that the concept provokes you to take a longer look at a player who steps into a meaningful role after another player on his team gets hurt, but what we can take away from this study is that minutes played and turnovers are the secondary metrics that have the highest correlation with player rater scores this year.
Devin Jordan is obsessed with statistical analysis, non-fiction literature, and electronic music. If you enjoyed reading him, follow him on Twitter
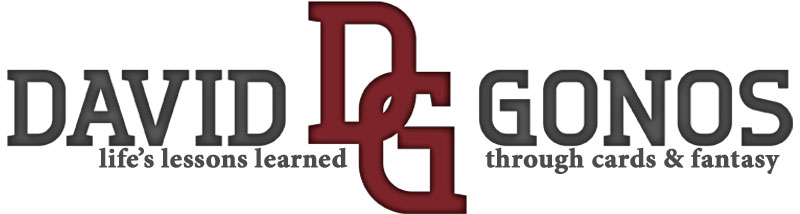